#Object classification through a single-pixel detector
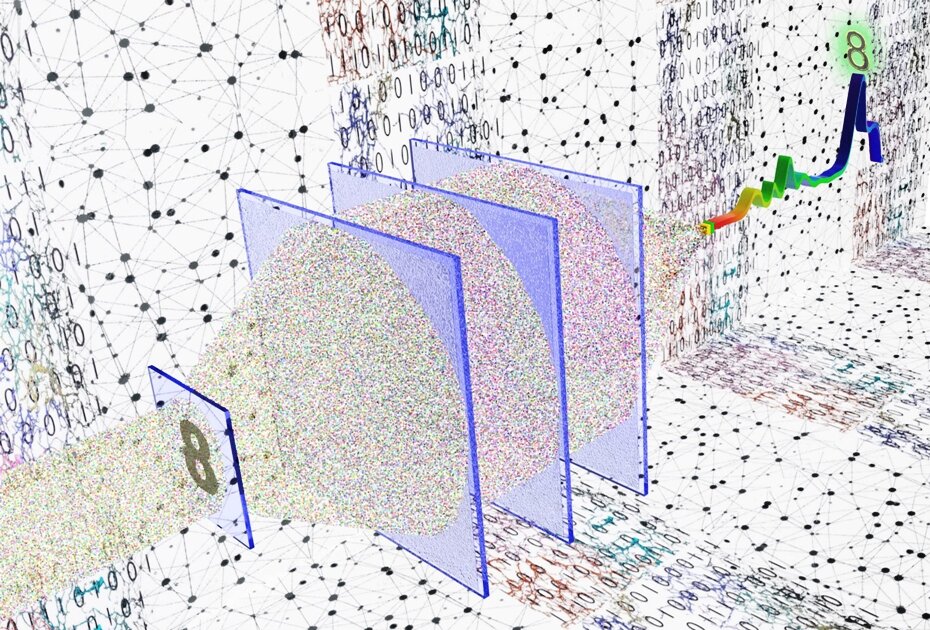
“#Object classification through a single-pixel detector”
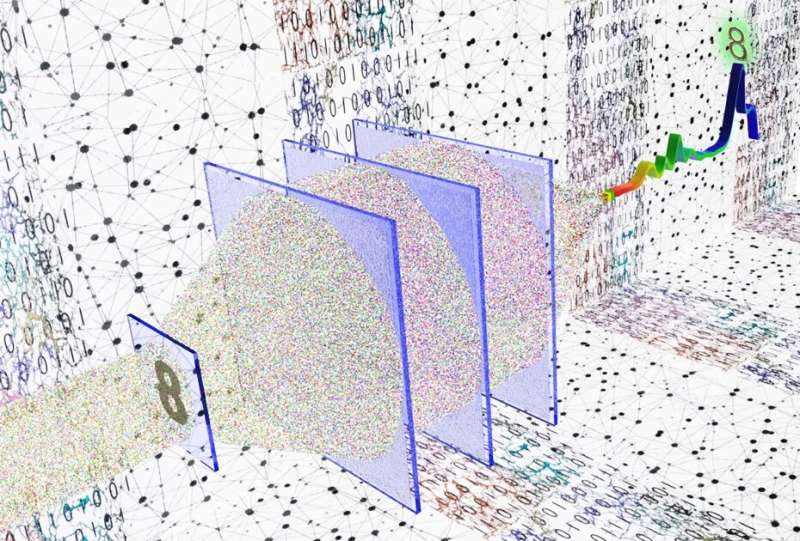
Machine vision systems have many applications, including self-driving cars, intelligent manufacturing, robotic surgery and biomedical imaging, among many others. Most of these machine vision systems use lens-based cameras, and after an image or video is captured, typically with a few megapixels per frame, a digital processor is used to perform machine-learning tasks, such as object classification and scene segmentation. Such a traditional machine vision architecture suffers from several drawbacks. First, the large amount of digital information makes it hard to achieve image/video analysis at high speed, especially using mobile and battery-powered devices. In addition, the captured images usually contain redundant information, which overwhelms the digital processor with a high computational burden, creating inefficiencies in terms of power and memory requirements. Moreover, beyond the visible wavelengths of light, fabricating high-pixel-count image sensors, such as what we have in our mobile phone cameras, is challenging and expensive, which limits the applications of standard machine vision methods at longer wavelengths, such as terahertz part of the spectrum.
UCLA researchers have reported a new, single-pixel machine vision framework that provides a solution to mitigate the shortcomings and inefficiencies of traditional machine vision systems. They leveraged deep learning to design optical networks created by successive diffractive surfaces to perform computation and statistical inference as the input light passes through these specially designed and 3D-fabricated layers. Unlike regular lens-based cameras, these diffractive optical networks are designed to process the incoming light at selected wavelengths with the goal of extracting and encoding the spatial features of an input object onto the spectrum of the diffracted light, which is collected by a single-pixel detector. Different object types or classes of data are assigned to different wavelengths of light. The input objects are automatically classified optically, merely using the output spectrum detected by a single pixel, bypassing the need for an image sensor-array or a digital processor. This all-optical inference and machine vision capability through a single-pixel detector that is coupled to a diffractive network provides transformative advantages in terms of frame rate, memory requirement and power efficiency, which are especially important for mobile computing applications.
In a study published in Science Advances, UCLA researchers experimentally demonstrated the success of their framework at terahertz wavelengths by classifying the images of handwritten digits using a single pixel detector and 3D printed diffractive layers. The optical classification of the input objects (handwritten digits) was performed based on the maximum signal among the ten wavelengths that were, one by one, assigned to different handwritten digits (0 through 9). Despite using a single-pixel detector, an optical classification accuracy of more than 96% was achieved. An experimental proof-of-concept study with 3D-printed diffractive layers showed a close agreement with the numerical simulations, demonstrating the efficacy of the single-pixel machine vision framework for building low-latency and resource-efficient machine learning systems. In addition to object classification, the researchers also connected the same single-pixel diffractive optical network with a simple, shallow electronic neural network, to rapidly reconstruct the images of the input objects based on only the power detected at ten distinct wavelengths, demonstrating task-specific image decompression.
This single-pixel object classification and image reconstruction framework could pave the way for the development of new machine vision systems that utilize spectral encoding of object information to achieve a specific inference task in a resource-efficient manner, with low-latency, low power and low pixel count. This new framework can also be extended to various spectral domain measurement systems, such as Optical Coherence Tomography, Infrared Spectroscopy and others, to create fundamentally new 3D imaging and sensing modalities integrated with diffractive network-based encoding of spectral and spatial information.
Jingxi Li et al. Spectrally encoded single-pixel machine vision using diffractive networks, Science Advances (2021). DOI: 10.1126/sciadv.abd7690
Citation:
Object classification through a single-pixel detector (2021, March 29)
retrieved 29 March 2021
from https://phys.org/news/2021-03-classification-single-pixel-detector.html
This document is subject to copyright. Apart from any fair dealing for the purpose of private study or research, no
part may be reproduced without the written permission. The content is provided for information purposes only.
If you liked the article, do not forget to share it with your friends. Follow us on Google News too, click on the star and choose us from your favorites.
For forums sites go to Forum.BuradaBiliyorum.Com
If you want to read more Like this articles, you can visit our Science category.