#Workplace AI will get hella boring before it becomes life-changing
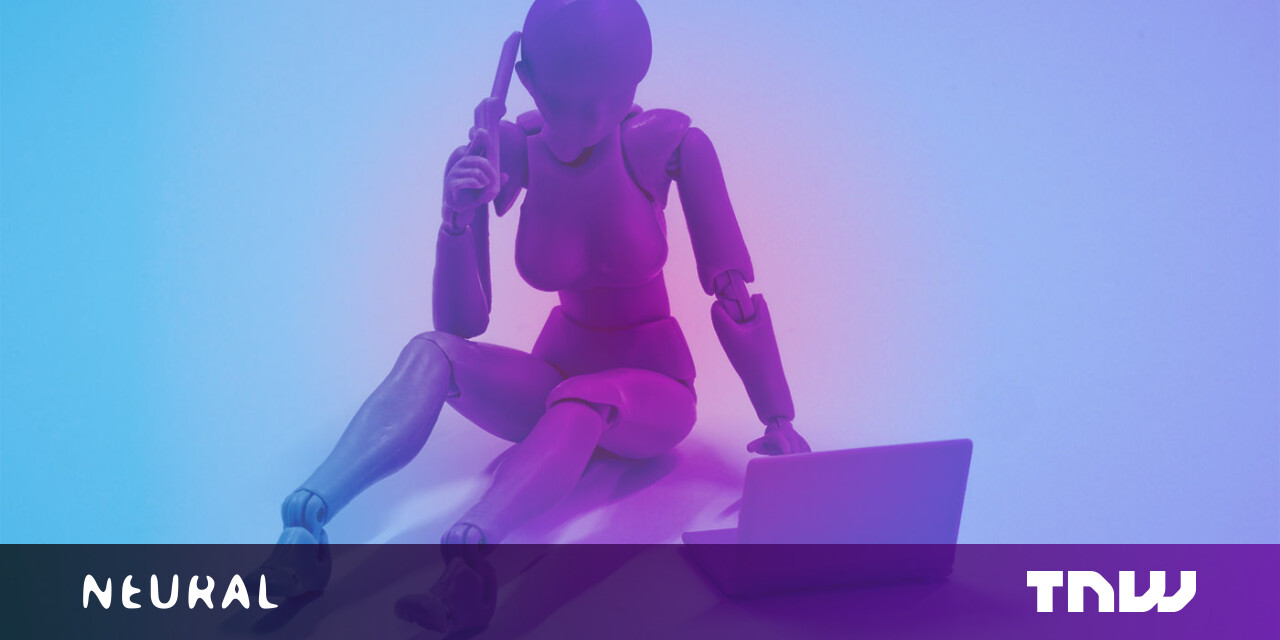
Table of Contents
“#Workplace AI will get hella boring before it becomes life-changing”
Digital technologies, and at their forefront artificial intelligence, are triggering fundamental shifts in society, politics, education, economy, and other fundamental aspects of life. These changes provide opportunities for unprecedented growth across different sectors of the economy. But at the same time, they entail challenges that organizations must overcome before they can tap into their full potential.
In a recent talk at an online conference organized by Stanford Human-Centered Artificial Intelligence (HAI), Stanford professor Erik Brynjolfsson discussed some of these opportunities and challenges.
Brynjolfsson, who directs Stanford’s Digital Economy Lab, believes that in the coming decade, the use of artificial intelligence will be much more widespread than it is today. But its adoption will also face a period of lull, also known as the J-curve.
“There’s a growing gap between what the technology is capable of and what it is already doing versus how we are responding to that,” Brynjolfsson says. “And that’s where a lot of our society’s biggest challenges and problems and some of our biggest opportunities lie.”
Machine learning and higher productivity
According to Brynjolfsson, the next decade will see significantly higher productivity thanks to a wave of powerful technologies—especially machine learning—that are finding their way into every computing device and application.
Advances in computer vision have been tremendous, especially in areas such as image recognition and medical imaging. Talking to phones, watches, and smart speakers has become commonplace thanks to advances in natural language processing and speech recognition. Product recommendation, ad placement, insurance underwriting, loan approval, and many other applications have benefited immensely from advances in machine learning.
In many areas, machine learning is reducing costs and accelerating production. For example, the application of large language models in programming can help software developers become much more productive and achieve more in less time.
In other areas, machine learning can help create applications that did not exist before. For example, generative deep learning models are creating new applications for arts, music, and other creative work. In areas such as online shopping, advances in machine learning can create major shifts in business models, such as moving from “shopping-then-shipping” to “shipping-then-shopping.”
The lockdowns and urgency caused by the covid-19 pandemic accelerated the adoption of these technologies in different sectors, including remote work tools, robotic process automation, powered drug research, and factory automation.
“The pandemic has been horrific in so many ways, but another thing it’s done is it’s accelerated the digitization of the economy, compressing in about 20 weeks what would have taken maybe 20 years of digitization,” Brynjolfsson says. “We’ve all invested in technologies that are allowing us to adapt to a more digital world. We’re not going to stay as remote as we are now, but we’re not going all the way back either. And that increased digitization of business processes and skills compresses the timeframe for us to adopt these new ways of working and ultimately drive higher productivity.”
The J-curve
The productivity potential of machine learning technologies has one big caveat.
“Historically, when these new technologies become available, they don’t immediately translate into productivity growth. Often there’s a period where productivity declines, where there’s a lull,” Brynjolfsson says. “And the reason there’s this lull is that you need to reinvent your organizations, you need to develop new business processes.”
Brynjolfsson calls this the “Productivity J-Curve” and has documented it in a paper published in the American Economic Journal: Macroeconomics. Basically, the great potential caused by new general-purpose technologies like the steam engine, electricity, and more recently machine learning requires fundamental changes in business processes and workflows, the co-invention of new products and business models, and investment in human capital.
These investments and changes often take several years, and during this period, they don’t yield tangible results. During this phase, the companies are creating “intangible assets,” according to Brynjolfsson. For example, they might be training and reskilling their workforce to employ these new technologies. They might be redesigning their factories or instrumenting them with new sensor technologies to take advantage of machine learning models. They might need to revamp their data infrastructure and create data lakes on which they can train and run ML models.
These efforts might cost millions of dollars (or billions in the case of large corporations) and make no change in the company’s output in the short term. At first glance, it seems that costs are increasing without any return on investment. When these changes reach their turning point, they result in a sudden increase in productivity.
AI J-curve

“We’re in this period right now where we’re making a lot of that painful transition, restructuring work, and there’s a lot of companies that are struggling with that,” Brynjolfsson says. “But we’re working through that, and these J-curves will lead to higher productivity—according to our research, we’re near the bottom and turning up.”
Making the transition to AI
Unfortunately, adapting to AI and other new digital technologies does not run on a predictable path. Most firms aren’t making the transition correctly or lack the creativity and understanding to make the transition. Various studies show that most applied machine learning projects fail.
“Only about the top 10-15 percent of firms are doing most of the investment in these intangibles. The other 85-90 percent of firms are lagging behind and are hardly making any of these restructuring needed,” Brynjolfsson says. “This is not just the big tech firms. This is within every industry, manufacturing, retail, finance, resources. In each category, we’re seeing the leading firms pulling away from the rest. There’s a growing performance gap.”
But while adopting new technologies is going to be difficult, it is happening at a much faster pace in comparison to previous cycles of technological advances because we are better prepared to make the transition.
“I think what is becoming clear is that it’s going to happen a lot faster in part because we have a much more professional class of people trying to study what works and what doesn’t work,” Brynjolfsson says. “Some of them are in business schools and academia. A lot of them are in consulting companies. Some of them are journalists. And there are people who are describing which practices work and which don’t.”
Another element that can help immensely is the availability of machine learning and data science tools to process and study the huge amounts of data available on organizations, people, and the economy.
For example, Brynjolfsson and his colleagues are working on a big dataset of 200 million job postings, which include the full text of the job description along with other information. Using different machine learning models and natural language processing techniques, they can transform the job posts into numerical vectors that can then be used for various tasks.
“We think of all the jobs as this mathematical space. We can understand how they can relate to each other,” Brynjolfsson says.
For example, they can make simple inferences such as how similar or different two or more job posts are based on their text descriptions. They can use other techniques such as clustering and graph neural networks to draw more important conclusions such as what kind of skills are more in demand, or how would the characteristics of a job post change if you modified the description to add AI skills such as Python or TensorFlow. Companies can use these models to find holes in their hiring strategies or to analyze the hiring decisions of their competitors and leading organizations.
“Those kinds of tools just didn’t exist as recently as five years ago, and I think it’s a revolution that is just as important as the microscope or some of the other revolutions in science,” Brynjolfsson says. “We now have them for social sciences and business to have this kind of visibility. That’s allowing us to make a transition a lot more rapidly than before.”
However, Brynjolfsson warns that not many companies are using these kinds of tools. This is perhaps further testament to his previous point that companies have not yet figured out the right transition strategy and are relying on old methods to restructure and adapt themselves to the age of AI. And at the center of this strategy should be the correct use of human capital.
“You have hundreds of billions of dollars of human capital, of skills walking out the door, and then the company tries to hire back people with the skills that they need. What they don’t realize is that the workers that they let go often had skills that were very adjacent to the ones they’re hiring for,” Brynjolfsson says.
With the help of machine learning, they will have better visibility and knowledge of their “skill adjacencies,” Brynjolfsson says. For example, a company might discover that instead of laying off a bunch of people and looking to hire new talent, perhaps all they need to do is a little bit of retraining and repurposing of their workforce.
“It’s much more expensive to hire somebody fresh than would have been for them to take some of those people who are already in the company and say, if we teach you Python or customer service skills or other skills, you can be doing this job that we’re looking to hire people for,” Brynjolfsson says. “My hope is that, in the coming decade, workers will be in a much better position to take full advantage of their capabilities and skills. And it will be good for the companies too to understand all the assets that they have in there, and machine learning can help a lot with understanding those relationships.”
This article was originally published by Ben Dickson on TechTalks, a publication that examines trends in technology, how they affect the way we live and do business, and the problems they solve. But we also discuss the evil side of technology, the darker implications of new tech, and what we need to look out for. You can read the original article here.
If you liked the article, do not forget to share it with your friends. Follow us on Google News too, click on the star and choose us from your favorites.
For forums sites go to Forum.BuradaBiliyorum.Com
If you want to read more like this article, you can visit our Technology category.