#Neuroscientists use AI to simulate how the brain makes sense of the visual world
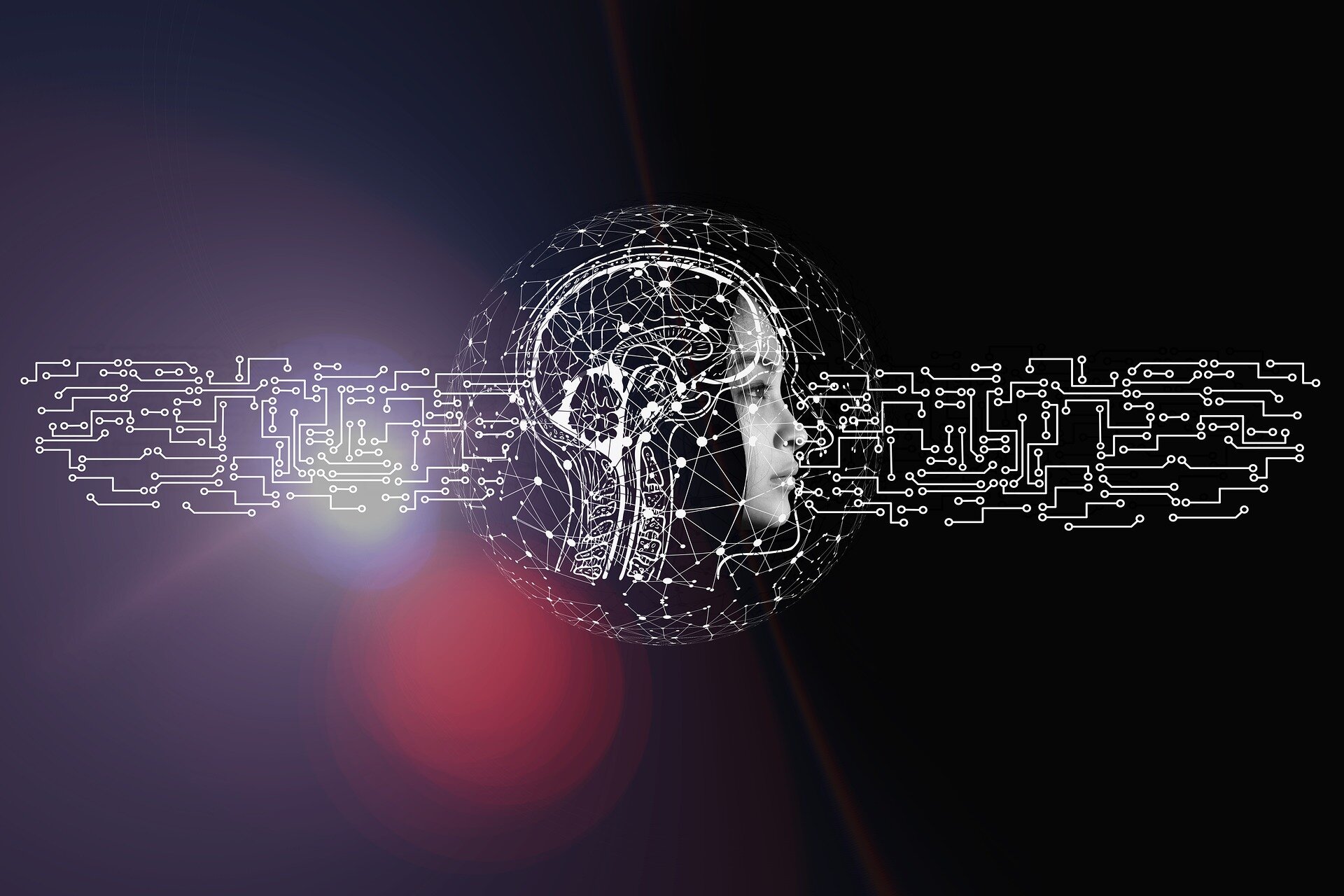
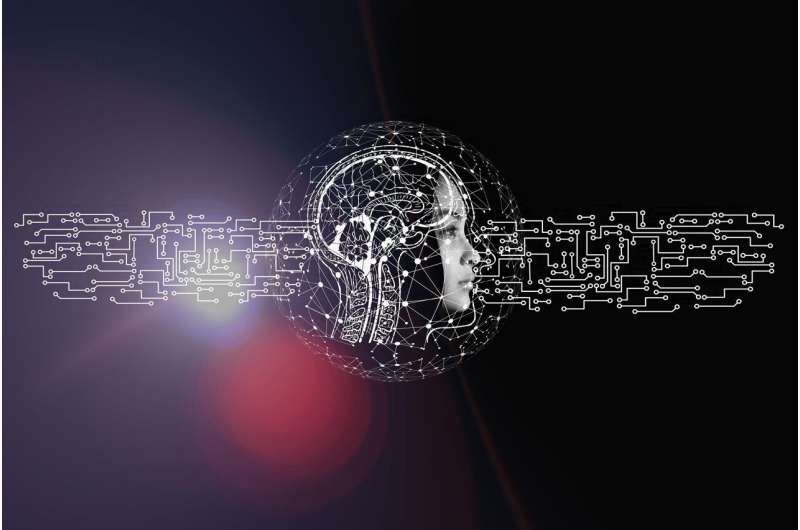
A research team at Stanford’s Wu Tsai Neurosciences Institute has made a major stride in using AI to replicate how the brain organizes sensory information to make sense of the world, opening up new frontiers for virtual neuroscience.
Watch the seconds tick by on a clock and, in visual regions of your brain, neighboring groups of angle-selective neurons will fire in sequence as the second hand sweeps around the clock face. These cells form beautiful “pinwheel” maps, with each segment representing a visual perception of a different angle. Other visual areas of the brain contain maps of more complex and abstract visual features, such as the distinction between images of familiar faces vs. places, which activate distinct neural “neighborhoods.”
Such functional maps can be found across the brain, both delighting and confounding neuroscientists, who have long wondered why the brain should have evolved a map-like layout that only modern science can observe.
To address this question, the Stanford team developed a new kind of AI algorithm—a topographic deep artificial neural network (TDANN)—that uses just two rules: naturalistic sensory inputs and spatial constraints on connections; and found that it successfully predicts both the sensory responses and spatial organization of multiple parts of the human brain’s visual system.
After seven years of extensive research, the findings were published in a new paper—”A unifying framework for functional organization in the early and higher ventral visual cortex”—on May 10 in the journal Neuron.
The research team was led by Wu Tsai Neurosciences Institute Faculty Scholar Dan Yamins, an assistant professor of psychology and computer science; and Institute affiliate Kalanit Grill-Spector, a professor in psychology.
Unlike conventional neural networks, the TDANN incorporates spatial constraints, arranging its virtual neurons on a two-dimensional “cortical sheet” and requiring nearby neurons to share similar responses to sensory input.
As the model learned to process images, this topographical structure caused it to form spatial maps, replicating how neurons in the brain organize themselves in response to visual stimuli. Specifically, the model replicated complex patterns such as the pinwheel structures in the primary visual cortex (V1) and the clusters of neurons in the higher ventral temporal cortex (VTC) that respond to categories like faces or places.
Eshed Margalit, the study’s lead author, who completed his Ph.D. working with Yamins and Grill-Spector, said the team used self-supervised learning approaches to help the accuracy of training models that simulate the brain.
“It’s probably more like how babies are learning about the visual world,” Margalit said. “I don’t think we initially expected it to have such a big impact on the accuracy of the trained models, but you really need to get the training task of the network right for it to be a good model of the brain.”
The fully trainable model will help neuroscientists better understand the rules of how the brain organizes itself, whether for vision, like in this study, or other sensory systems such as hearing.
“When the brain is trying to learn something about the world—like seeing two snapshots of a person—it puts neurons that respond similarly in proximity in the brain and maps form,” said Grill-Spector, who is the Susan S. and William H. Hindle Professor in the School of Humanities and Sciences. “We believe that principle should be translatable to other systems, as well.”
This innovative approach has significant implications for both neuroscience and artificial intelligence. For neuroscientists, the TDANN provides a new lens to study how the visual cortex develops and operates, potentially transforming treatments for neurological disorders. For AI, insights derived from the brain’s organization can lead to more sophisticated visual processing systems, akin to teaching computers to ‘see’ as humans do.
The findings could also help explain how the human brain operates with such stellar energy efficiency. For example, the human brain can compute a billion-billion math operations with only 20 watts of power, compared with a supercomputer that requires a million times more energy to do the same math.
The new findings emphasize that neuronal maps—and the spatial or topographic constraints that drive them—likely serve to keep the wiring connecting the brain’s 100 billion neurons as simple as possible. These insights could be key to designing more efficient artificial systems inspired by the elegance of the brain.
“AI is constrained by power,” Yamins said. “In the long run, if people knew how to run artificial systems at a much lower power consumption, that could fuel AI’s development.”
More energy-efficient AI could help grow virtual neuroscience, where experiments could be done more quickly and at a larger scale. In their study, the researchers demonstrated as a proof of principle that their topographical deep artificial neural network reproduced brain-like responses to a wide range of naturalistic visual stimuli, suggesting that such systems could, in the future, be used as fast, inexpensive playgrounds for prototyping neuroscience experiments and rapidly identifying hypotheses for future testing.
Virtual neuroscience experiments could also advance human medical care. For example, better training an artificial visual system in the same way a baby visually learns about the world might help an AI see the world like a human, where the center of gaze is sharper than the rest of a field of view. Another application could help develop prosthetics for vision or simulate exactly how diseases and injuries affect parts of the brain.
“If you can do things like make predictions that are going to help develop prosthetic devices for people that have lost vision, I think that’s really going to be an amazing thing,” Grill-Spector said.
Eshed Margalit et al, A unifying framework for functional organization in early and higher ventral visual cortex, Neuron (2024). DOI: 10.1016/j.neuron.2024.04.018
Citation:
Neuroscientists use AI to simulate how the brain makes sense of the visual world (2024, May 28)
retrieved 28 May 2024
from https://medicalxpress.com/news/2024-05-neuroscientists-ai-simulate-brain-visual.html
This document is subject to copyright. Apart from any fair dealing for the purpose of private study or research, no
part may be reproduced without the written permission. The content is provided for information purposes only.
If you liked the article, do not forget to share it with your friends. Follow us on Google News too, click on the star and choose us from your favorites.
If you want to read more Like this articles, you can visit our Science category.