#Automated total root length estimation from in situ images without segmentation
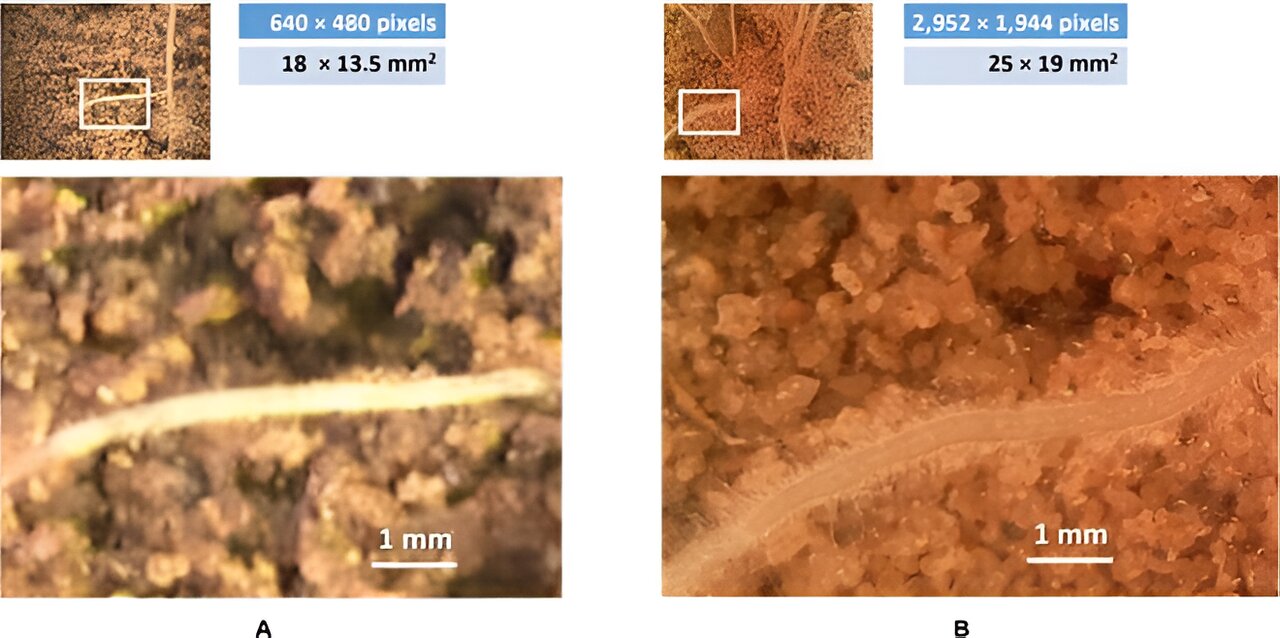
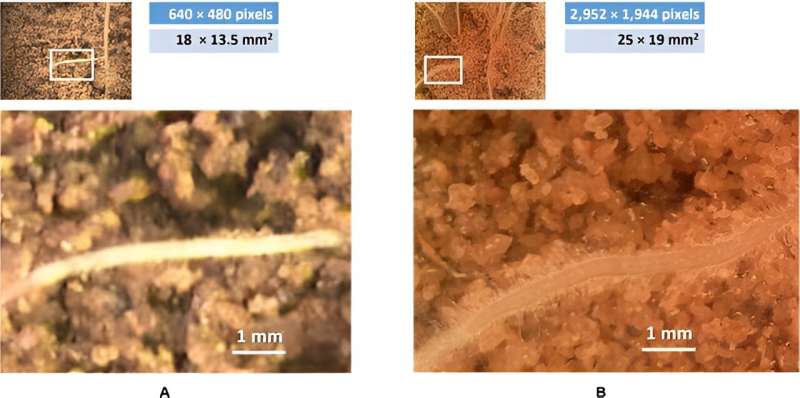
Climate change stresses severely limit crop yields, with root traits playing a vital role in stress tolerance, thus highlighting the importance of root phenotyping for crop improvement. Recent advances in image-based root phenotyping, particularly through the minirhizotron (MR) technique, offer insights into root dynamics under stress. However, the manual and subjective nature of MR image analysis poses significant challenges.
This highlights the need for automated imaging systems and tools to streamline and objectify the process, enhancing the efficiency and objectivity of root phenotyping.
In January 2024, Plant Phenomics published a research article titled “Automatic Root Length Estimation from Images Acquired In Situ without Segmentation.” This study advances the field of root phenotyping by adapting convolutional neural network-based models for estimating total root length (TRL) from MR images without the need for segmentation.
Utilizing manual annotations from Rootfly software, researchers explored a regression-based model and a detection-based model that identifies annotated root points, with the latter offering a visual inspection capability of MR images.
The models were rigorously tested across 4,015 images from diverse crop species under varied abiotic stresses, demonstrating high accuracy (R2 values between 0.929 and 0.986) in TRL estimation compared to manual measurements. This accuracy underscores the potential of our approach to significantly enhance root phenotyping’s efficiency and reliability.
The study’s results indicate that the detection-based model generally outperforms the regression model, particularly in challenging datasets, by incorporating additional root coordinate information. This finding is critical for high-quality image datasets, where automated TRL estimation remains robust.
Moreover, researchers conducted a sensitivity analysis to highlight the impact of image quality and dataset size on model performance, revealing the significant influence of image quality. The models’ ability to differentiate between images with and without roots, with a minimal error margin, further illustrates their practical utility in precision agriculture by enabling real-time monitoring of root growth.
The analysis was then extended to evaluate root length density (RLD) calculations, demonstrating the models’ effectiveness in capturing root distribution patterns in soil, which is vital for understanding water and nutrient extraction. The models’ capability to track root dynamics over timeâincluding the identification of root disappearanceâhighlights their potential to inform timely agricultural decisions regarding water and nutrient management.
In conclusion, this research presents a groundbreaking approach to root phenotyping, offering robust, automated tools for TRL estimation from MR images, thereby facilitating the rapid and accurate assessment of root growth patterns. This advancement holds significant promise for enhancing precision agriculture practices, enabling growers to make informed decisions based on detailed root growth information.
Faina Khoroshevsky et al, Automatic Root Length Estimation from Images Acquired In Situ without Segmentation, Plant Phenomics (2023). DOI: 10.34133/plantphenomics.0132
Citation:
Root phenotyping research: Automated total root length estimation from in situ images without segmentation (2024, March 11)
retrieved 11 March 2024
from https://phys.org/news/2024-03-root-phenotyping-automated-total-length.html
This document is subject to copyright. Apart from any fair dealing for the purpose of private study or research, no
part may be reproduced without the written permission. The content is provided for information purposes only.
If you liked the article, do not forget to share it with your friends. Follow us on Google News too, click on the star and choose us from your favorites.
If you want to read more Like this articles, you can visit our Science category.